Recognizability bias in citizen science photographs
Koch, Wouter; Hogeweg, Laurens; Nilsen, Erlend Birkeland; O'Hara, Robert B.; Finstad, Anders Gravbrøt
Peer reviewed, Journal article
Published version
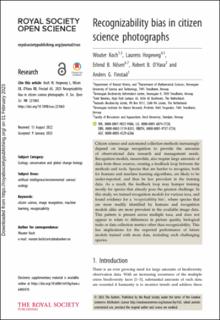
View/ Open
Date
2023Metadata
Show full item recordCollections
Original version
Koch, W., Hogeweg, L., Nilsen, E. B., O'Hara, R. B.& Finstad, A. G. (2023). Recognizability bias in citizen science photographs, 10(2), Article 221063. doi: 10.1098/rsos.221063Abstract
Citizen science and automated collection methods increasinglydepend on image recognition to provide the amountsof observational data research and management needs.Recognition models, meanwhile, also require large amounts ofdata from these sources, creating a feedback loop between themethods and tools. Species that are harder to recognize, bothfor humans and machine learning algorithms, are likely to beunder-reported, and thus be less prevalent in the trainingdata. As a result, the feedback loop may hamper trainingmostly for species that already pose the greatest challenge. Inthis study, we trained recognition models for various taxa, andfound evidence for a‘recognizability bias’, where species thatare more readily identified by humans and recognitionmodels alike are more prevalent in the available image data.This pattern is present across multiple taxa, and does notappear to relate to differences in picture quality, biologicaltraits or data collection metrics other than recognizability. Thishas implications for the expected performance of futuremodels trained with more data, including such challenging species. citizen science, image recognition, machinelearning, recognizability, artificial intelligence/environmental science/ecology, Ecology, conservation and global change biology